Bioinformatics: Why It Matters
- 16th July 2018
- Posted by: Claudine Gabriele
- Category: Articles
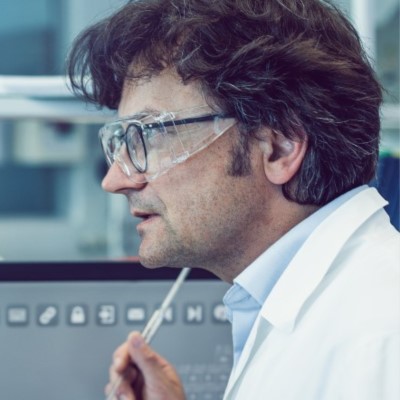
Thousands of data points are generated by ‘omics experiments. These can be fragmented and come from different sources in a range of environments – and they all need to be analysed. Large datasets can be unwieldly without the expertise to deal with them, both from analytical and contextual standpoints. This is where bioinformatics, and bioinformaticians, can come in. Bioinformatics applies computer-based approaches to the understanding of biological processes, which are key when looking at the genome and other ‘omics data.
We’ve listed the top three reasons that you need bioinformatics:
1. Expertise of analysis
Biological research has a context. A computer-based approach is now vital for larger-scale datasets, with the context that the data comes from still necessary to fully understand it. Bioinformatics can study entire genomes, and snapshots of many genes at one time, instead of only one by one – allowing for trends to be identified.
Genomes are large – the human genome has just under 19,000 genes made up of three billion base pairs of DNA. In comparison, the mosquito genome has 280 million base pairs making up 14,000 genes, while even the nematode worm genome has 100 million base pairs for 19,000 genes. This means that the datasets for ‘omics data are huge – and need more expertise to be able to analyse them.
It’s not just genomics that makes up ‘omics data; transcriptomics for example, the study of when genes are turned on and off at a specific time, creates equally as vast data sets. The biology behind the data produced needs to be understood, to be able to give context to your results. Patterns and trends need to be identified and can then be associated with particular diseases or drug responses, allowing your data to become more useful and give clear actions or information for the future.
2. Reproducible workflows
With large datasets, you need to ensure that the answers you get are correct every time a calculation is run. To do this, bioinformaticians use standardised pipelines that are created so data can be processed repeatedly to get the same answers – enabling you to make a consistent decision that is based on your data.
The same general steps are taken, no matter the data type received. Quality control is the first and most important step in any pipeline. This ensures that the data is able to be processed and moved further down the pipeline, and no unexpected errors will crop up later in the analysis.
With datasets that run into the millions (and potentially even billions), data analysis can take days. By having analysis workflows set up, combined with the background knowledge to interpret the data, the analysis of large datasets can be streamlined, meaning that your study isn’t held up unnecessarily while data analysis takes place.
3. Integration of datatypes
Data from ‘omics studies is rarely just one type. In clinical trials specifically, data can be collected from a variety of different sources. It can be received as patient demographic information, outcome data, various types of images, as well as specifically ‘omics data. There is a need to integrate all of these data types for analysis – to make sure they are analysed together as they are all relevant to each other.
Integration of different data types and then analysing them together allows for the genetic basis of diseases or drug responses to be better understood. Outcome data can be linked to the ‘omics data collected, clearly showing where in the genome a disease affects, or how a specific drug causes a response.
For more information on how your research can benefit from bioinformatics and where Fios can help, watch our video:
Leave a Reply
You must be logged in to post a comment.