Multiple Data Type Integration
- 24th November 2020
- Posted by: Claudine Gabriele
- Categories: Articles, Bioinformatics, Biomarkers, Gene Expression Analysis, Mass Spectrometry, Proteomics, Single Cell Analysis
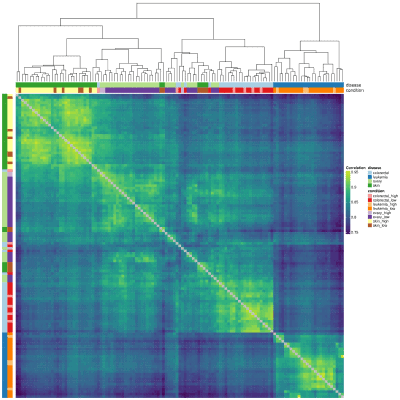
In many studies, more than one type of data will be generated. From combining gene expression data with patient metadata, what is the benefit of generating and integrating multiple data types?
What types of projects can use integrated data?
Many types of projects integrate data from different sources for analysis. Both preclinical and clinical stages of drug development can generate many types of data.
In preclinical development, in both early and later stages, projects may focus on identifying molecular markers or biomarkers that are associated with specific outcomes including sensitivity. Gene expression changes can also be combined along with copy number variant (CNV) and mutational data to identify biomarkers that are indicative of a compound’s sensitivity. Later stages of preclinical development where animal models are in use can also assess gene expression changes with these types of data.
Moving into clinical trials and studies, all phases of trials can make use of integrated analysis. Integration of different data types can help to assess safety, tolerability, and efficacy for patients, and is not limited to one area of therapeutic study. Proteomics data can be combined with pharmacokinetics (PK) data to assess patient outcomes after treatment and relate response to exposure. Clinical data such as overall survival and progression-free survival can also be integrated with biomarker and other ‘omics data generated through the course of a trial to better explore the effects of a potential drug on patients and their overall response. Those biomarkers can potentially be used to stratify patients in further trials.
What types of data can be integrated?
Nearly all types of biological data can be integrated together for analysis. The data can also be sourced from the public domain – for example, our bioinformatics analysis can combine in-house generated data with data mined from a range of public sources.
We have worked on projects that have combined:
- Single nucleotide variants (SNVs), indels, and CNVs.
- Gene expression, CNV, and mutation data.
- Biomarkers with clinical factors (e.g. age, sex, clinical data).
- Gene expression and PK/PD markers.
- Gene expression data from both blood and gut biopsies with PK data from blood and stool samples.
- Sensitivity with cell line profiling data. Gene expression, CNV, and mutational data were also integrated with the sensitivity data.
- Tumour mutational burden and clinical response, along with patients’ individual mutations, SNVs, indels and CNVs.
- Clinical information (such as overall survival and progression-free survival) alongside biomarker assessment.
- Pharmacogenomic (PGx data) combined with PK, and clinical information (such as body mass index (BMI) and liver function tests).
Additionally, all therapeutic areas of research are able to generate and integrate multiple data sources. Fios Genomics’ publications page holds many examples of integrated analysis work for a variety of disease areas.
Benefits of integrating multiple data types
By unifying the view of impact from a drug through integration of multiple data types, such as clinical, ‘omics, or biomarkers, you gain a comprehensive understanding of its effect on a biological system, whether cell model or patient. Including patient-specific data, such as their clinical and genetic data, can show how a disease has progressed on an individual basis which can lead to the development of more precise therapeutics.
The effect that a novel therapeutic has is not one-dimensional. Through data integration, you can build a more detailed picture of the effect that your compound or therapeutic has – whether your research is preclinical, where outcomes or changes will be found in cell or animal models, or clinical with patient trials. This is not without computational challenges however; as different data is generated in different formats, these might need careful conversion before integration.
Interested in learning more?
Our bioinformatic analysis report ‘Expression, copy number and SNP analysis of the Broad Avana CRISPR and DepMap 19Q2 CCLE data and BRAF gene dependency’ describes the integration of gene expression, copy number and mutation datasets from the Broad Institute Cancer Cell Line Encyclopaedia (CCLE), with BRAF gene dependency data from the Broad Institute Avana Achilles project. The aim was to identify genetic features that were significantly associated with BRAF gene dependency across the DepMap cancer cell lines and indications. This approach highlighted biomarkers of BRAF dependency and could help prioritise potential novel therapeutic targets. Access our sample report now.
Read more
Methods for biological data integration: perspectives and challenges
Leave a Reply
You must be logged in to post a comment.